There are several recruitment companies that specialize in the technology sector, including big data. They focus on matching professionals with expertise in analytics, data science, machine learning, and artificial intelligence with companies in need of their skills. Here are some types of agencies that might specialize in big data:
- Tech-Specific Recruitment Agencies: These are firms that focus exclusively on the technology sector and often have divisions or specialists dedicated to data roles.
- Executive Search Firms: These firms typically look for high-level professionals and may have a focus on senior data science and big data roles.
- Staffing Agencies with IT Focus: General staffing agencies often have IT departments that understand the nuances of recruiting for big data positions.
- Boutique Agencies: Smaller, niche recruitment agencies can offer a more personalized service and have deep networks within the big data field.
When searching for a recruitment company to assist you in finding a position in big data, consider the following:
- Expertise in Big Data: Look for agencies that have a proven track record of placing candidates in big data roles. - Industry Knowledge: It's beneficial if the agency is well-versed in the specific industries where big data skills are in high demand, such as finance, healthcare, retail, or technology. - Client Portfolio: Consider the types of companies the agency works with. Are they startups, mid-size companies, or large corporations? - Candidate Support: Good recruitment firms offer resume advice, interview coaching, and detailed job market insights.
- Geographical Coverage: Some recruitment companies have a global reach, which can be beneficial if you are open to relocating.
It's a good practice to research and connect with a few different firms to find the one that best matches your career aspirations and can provide the level of support you need. You can often find this information through a recruitment company's website, industry networking events, or recommendations from professionals within your network.
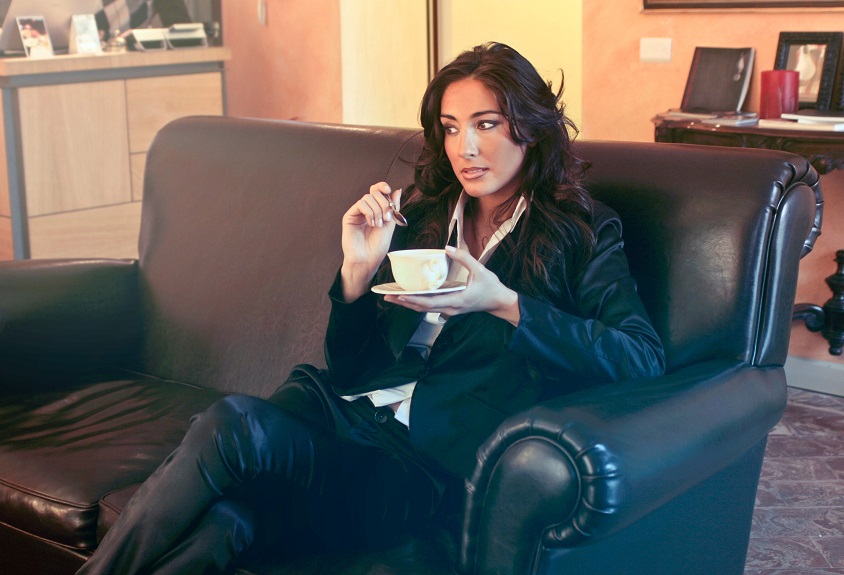
Is Big Data Always Training AI?
No, big data isn't always used for training artificial intelligence (AI), but it is one of the significant uses. Big data refers to the large volumes of data that businesses and organizations collect, which can be structured or unstructured and come from various sources. The relationship between big data and AI is strong because AI systems require vast amounts of data to learn and make informed decisions. However, big data has a range of other uses beyond AI:
Uses of Big Data:
- Analytics and Insights: Organizations use big data to uncover patterns, trends, and associations, especially relating to human behavior and interactions, which can inform business decisions.
- Business Intelligence (BI): Big data powers BI tools that help companies make strategic decisions by analyzing current and historical data within the business context.
- Risk Management: Big data enables companies to model various scenarios and predict outcomes, which is crucial for risk management and contingency planning.
- Personalization: Retailers and service providers use big data to tailor their offerings to individual customers, enhancing the customer experience and improving sales.
- Operational Efficiency: By analyzing big data, organizations can identify inefficiencies and bottlenecks in their operations and work to improve them.
- Product Development: Insights from big data analysis can guide the development of new products or the improvement of existing ones.
- Healthcare: In healthcare, big data is used for predicting epidemics, improving quality of life, avoiding preventable deaths, and reducing healthcare costs by identifying patterns and treatment outcomes.
Uses of Big Data in AI:
- Machine Learning Models: Big data is used to train machine learning models, which can improve over time as they are exposed to more data.
- Natural Language Processing (NLP): AI applications like virtual assistants use big data to understand and interpret human language more effectively.
- Computer Vision: AI systems that interpret visual data require large datasets of images and videos to accurately recognize and classify visual objects.
- Predictive Analytics: AI uses big data to forecast future trends and behaviors, which is particularly useful in fields like finance, marketing, and healthcare.
In summary, while big data is integral to AI development, its applications are diverse and extend far beyond the realm of AI. The utilization of big data spans across various domains where the analysis of large and complex datasets is needed to drive decisions and innovations.

Would that First List Typically Incorporate AI as Part of the Analysis?
The first list of big data uses that I mentioned often does incorporate AI and machine learning as part of the analysis process, but not necessarily. Here's a breakdown of how AI might be involved:
- Analytics and Insights: AI can be used to automate the extraction of insights from big data, using machine learning algorithms to identify patterns and trends that might not be apparent to human analysts.
- Business Intelligence (BI): Modern BI tools frequently include AI components to provide more advanced predictive analytics and to handle the large volumes of data more efficiently.
- Risk Management: AI models can help in predicting risks by analyzing large datasets to identify potential future issues, such as credit risk in banking or safety incidents in manufacturing.
- Personalization: AI is often at the heart of personalization, using algorithms to analyze customer data and provide recommendations or customized experiences.
- Operational Efficiency: AI can optimize operations by predicting and intelligently scheduling maintenance, managing supply chains, or optimizing routes for delivery.
- Product Development: AI can help in analyzing customer feedback and market trends to guide new product development.
- Healthcare: AI is used in predictive analytics for patient outcomes, in diagnostics through image recognition, and in personalizing patient treatment plans.
In many cases, AI enhances the capabilities of big data analytics by bringing in the ability to learn from data without being explicitly programmed. However, it's important to note that not every analysis of big data requires AI. Traditional statistical methods and data mining techniques are still widely used and can be very effective, especially when the analytical needs are straightforward or when interpretability is more important than the predictive power that AI models provide.
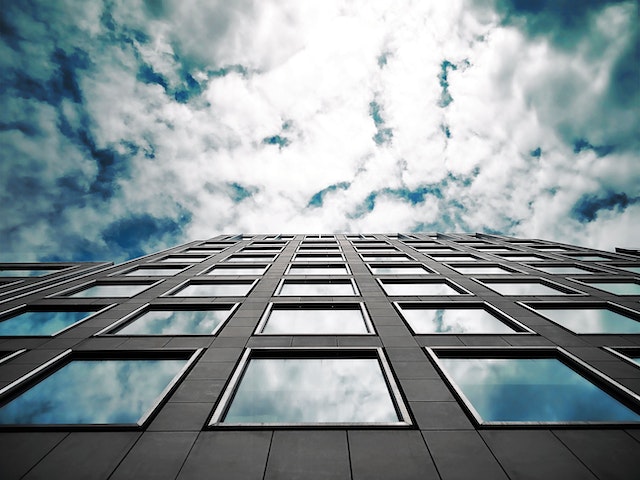
Would Machine Learning be the Skill that Big Data Recruiters are Looking for?
Yes, machine learning is a highly sought-after skill among big data recruiters. The ability to design, implement, and manage machine learning models is crucial for many roles that involve working with big data. Here are some reasons why machine learning expertise is in demand:
- Data-Driven Decision Making: Companies rely on machine learning models to make informed decisions based on large datasets, and they need professionals who can build and interpret these models.
- Predictive Analytics: Machine learning is fundamental for predictive analytics, where historical data is used to forecast future events, behaviors, and trends.
- Automation of Analytical Processes: Machine learning can automate complex data analysis processes, making it faster and more efficient to derive insights from big data.
- Innovative Product Features: Products that offer personalized recommendations or adapt to user behavior, such as streaming services or e-commerce platforms, often utilize machine learning algorithms.
- Operational Optimization: Machine learning can optimize various operational aspects, such as supply chain logistics, dynamic pricing, and inventory management.
- Advanced AI Applications: Machine learning is at the core of advanced AI applications, including natural language processing, computer vision, and speech recognition, all of which require analysis of large volumes of data.
Recruiters looking for big data professionals often seek candidates with a combination of skills, including but not limited to:
- Understanding of Machine Learning Algorithms: Knowing a range of algorithms and when to apply them is crucial. - Data Preprocessing: Ability to clean and organize data for analysis. - Programming Skills: Proficiency in languages such as Python, R, or Java. - Statistical Knowledge: A strong foundation in statistics is important for building and evaluating models. - Experience with Big Data Platforms: Familiarity with platforms like Apache Hadoop, Spark, or others used for large-scale data processing.
- Data Visualization and Communication: The ability to present findings clearly to non-technical stakeholders.
While machine learning is a key skill, it's often part of a broader set of skills that recruiters look for in candidates for big data roles. These skills include understanding data structures, database management, cloud computing, and often domain-specific knowledge depending on the industry.
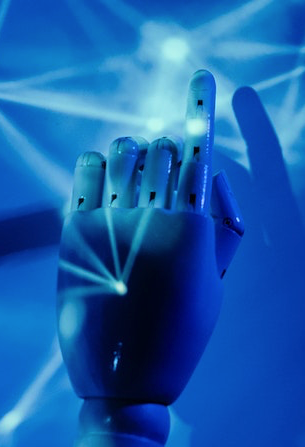
Are Big Data Programmers Being Replaced with AI Bots?
It has to be said, AI and machine learning technologies are certainly advancing rapidly, and some aspects of programming and data analysis can be automated with these tools. However, the complete replacement of big data programmers with AI bots is not a current reality for several reasons:
- Complex Problem-Solving: Big data programmers tackle complex, often novel problems that require human ingenuity and creativity. AI bots can support and enhance this work but are not yet capable of fully independent creative problem-solving.
- Understanding Context: Humans are still required to understand the broader context of the data, the business implications of analysis, and to make judgment calls that take into account factors outside the dataset.
- Interdisciplinary Communication: Programmers often need to collaborate with stakeholders from various parts of a business to understand requirements and constraints. Communication and negotiation skills are currently beyond the scope of AI bots.
- Ethical Considerations: Decisions about data usage, privacy, and ethical implications require human oversight. AI is not equipped to handle these considerations without human guidance.
- Customization and Integration: While AI can automate certain tasks, big data programmers are needed to customize solutions to fit specific organizational needs and integrate new tools with existing systems.
- Maintenance and Updates: AI systems themselves need maintenance, updating, and improvement over time, tasks that require skilled human programmers.
- AI Training and Supervision: AI bots, particularly those involved in machine learning, require initial training, continuous supervision, and adjustment by human experts.
- Quality Assurance and Error Correction: AI can help to identify errors or inefficiencies in code, but humans are still better at understanding whether something is truly an error or a necessary adaptation, and in creating fixes.
Automation and AI tools are being used to augment the capabilities of big data programmers, making certain tasks faster and more efficient. For example, AI can assist with code completion, bug detection, and even writing simple scripts. This allows programmers to focus on more strategic work that adds value to their organizations. In essence, AI bots are tools that can significantly enhance productivity but are not close to replacing big data programmers altogether. The role of the programmer is evolving, with a growing emphasis on overseeing and working alongside AI systems.